Data Analysis for Life Sciences 7: Case Studies in Functional Genomics - Harvard University
Course
Online
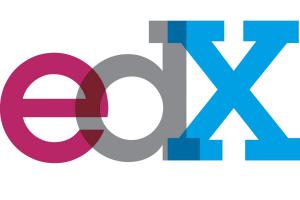
Description
-
Type
Course
-
Methodology
Online
-
Start date
Different dates available
Explore data analysis of several experimental protocols, using open source software, including R and Bioconductor.
Facilities
Location
Start date
Start date
About this course
PH525.3x, PH525.4x
Reviews
This centre's achievements
All courses are up to date
The average rating is higher than 3.7
More than 50 reviews in the last 12 months
This centre has featured on Emagister for 8 years
Subjects
- Data analysis
- IT
- Genomics
- Case Studies
- Biology
- R
- Bioconductor
Course programme
We will explain how to start with raw data, and perform the standard processing and normalization steps to get to the point where one can investigate relevant biological questions. Throughout the case studies, we will make use of exploratory plots to get a general overview of the shape of the data and the result of the experiment. We start withRNA-seq data analysis covering basic concepts of RNA-seq and a first look at FASTQ files. We will also go over quality control of FASTQ files; aligning RNA-seq reads; visualizing alignments and move on to analyzing RNA-seq at the gene-level: counting reads in genes; Exploratory Data Analysis and variance stabilization for counts; count-based differential expression; normalization and batch effects. Finally, we cover RNA-seq at the transcript-level: inferring expression of transcripts (i.e. alternative isoforms); differential exon usage. We will learn the basic steps in analyzing DNA methylation data, including reading the raw data, normalization, and finding regions of differential methylation across multiple samples. The course will end with a brief description of the basic steps for analyzing ChIP-seq datasets, from read alignment, to peak calling, and assessing differential binding patterns across multiple samples.
Given the diversity in educational background of our students we have divided the series into seven parts. You can take the entire series or individual courses that interest you. If you are a statistician you should consider skipping the first two or three courses, similarly, if you are biologists you should consider skipping some of the introductory biology lectures. Note that the statistics and programming aspects of the class ramp up in difficulty relatively quickly across the first three courses. By the third course will be teaching advanced statistical concepts such as hierarchical models and by the fourth advanced software engineering skills, such as parallel computing and reproducible research concepts.
- Mapping reads
- Quality assessment of Next Generation Data
- Analyzing RNA-seq data
- Analyzing DNA methylation data
- Analyzing ChIP Seq data
Additional information
Data Analysis for Life Sciences 7: Case Studies in Functional Genomics - Harvard University