Data Preprocessing For Machine Learning Using MATLAB
Course
Online
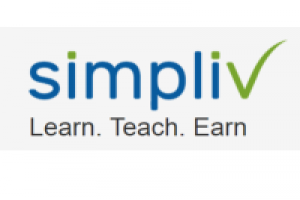
Description
-
Type
Course
-
Methodology
Online
-
Start date
Different dates available
Basic Course Description This course is for you if you want to fully equip yourself with the art of applied machine learning using MATLAB. This course is also for you if you want to apply the most commonly used data preprocessing techniques without having to learn all the complicated maths. Additionally, this course is also for you if you have had previous hours and hours of machine learning implementation but could never figure out how to further improve the peformance of the machine learning algorithms. By the end of this course, you will have at your fingertips, a vast variety of most commonly used data preprocessing techniques that you can use instantly to maximize your insight into your data set. The approach in this course is very practical and we will start everything from very scratch. We will immediately start coding after a couple of introductory tutorials and we try to keep the theory to bare minimal. All the coding will be done in MATLAB which is one of the fundamental programming languages for engineer and science students and is frequently used by top data science research groups world wide. Below is the brief outline of this course. Segment 1: Introduction to course and MATLAB
Segment 2: Handling Missing Values
Segment 3: Dealing with Categorical Variables
Segment 4: Outlier Detection
Segment 5: Feature Scaling and Data Discretization
Segment 6: Project: Selecting Techniques for your DatasetYour Benefits and Advantages: You will be sure of receiving quality contents from the instructors.
You have lifetime access to the course.
You have instant and free access to any updates i add to the course.
You have access to all Questions and discussions initiated by other students.
You will receive my support regarding any issues related to the course.
Check out the curriculum and Freely available lectures for a quick insight.It's time to take Action!Click the "Take This Course" button at the top right now!
.
.
Facilities
Location
Start date
Start date
About this course
How to effectively proprocess data before analysis
How to implement different preprocessing methods using matlab
Take away code templates for quickly preprocessing your data
Decide which method choose for your dataset
Students, Entrepreneurs, Researchers, Instructors, Engineers, Programmers, Simulators
Anyone who want to analyze the data
Reviews
This centre's achievements
All courses are up to date
The average rating is higher than 3.7
More than 50 reviews in the last 12 months
This centre has featured on Emagister for 4 years
Subjects
- Access
Course programme
Additional information
Data Preprocessing For Machine Learning Using MATLAB