Introduction to Probability - The Science of Uncertainty - Massachusetts Institute of Technology
Course
Online
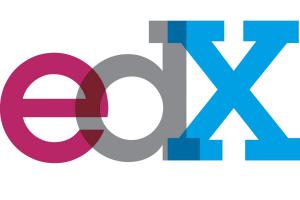
Description
-
Type
Course
-
Methodology
Online
-
Start date
Different dates available
An introduction to probabilistic models, including random processes and the basic elements of statistical inference.
Facilities
Location
Start date
Start date
About this course
College-level calculus (single-variable and multivariable). Although this is not a mathematics course, it does rely on the language and some tools from mathematics. It requires a level of comfort with mathematical reasoning, familiarity with sequences, limits, infinite series, the chain rule, as well as the ability to work with ordinary or multiple integrals.
Reviews
This centre's achievements
All courses are up to date
The average rating is higher than 3.7
More than 50 reviews in the last 12 months
This centre has featured on Emagister for 8 years
Subjects
- Probability
- Statistics
- Probabilistic models
- Statistical
- Probabilistic modeling
Course programme
The world is full of uncertainty: accidents, storms, unruly financial markets, noisy communications. The world is also full of data. Probabilistic modeling and the related field of statistical inference are the keys to analyzing data and making scientifically sound predictions.
Probabilistic models use the language of mathematics. But instead of relying on the traditional "theorem - proof" format, we develop the material in an intuitive -- but still rigorous and mathematically precise -- manner. Furthermore, while the applications are multiple and evident, we emphasize the basic concepts and methodologies that are universally applicable.
The course covers all of the basic probability concepts, including:
- multiple discrete or continuous random variables, expectations, and conditional distributions
- laws of large numbers
- the main tools of Bayesian inference methods
- an introduction to random processes (Poisson processes and Markov chains)
The contents of this course are essentially the same as those of the corresponding MIT class (Probabilistic Systems Analysis and Applied Probability) -- a course that has been offered and continuously refined over more than 50 years. It is a challenging class, but it will enable you to apply the tools of probability theory to real-world applications or your research.
- The basic structure and elements of probabilstic models
- Random variables, their distributions, means, and variances
- Probabilistic calculations
- Inference methods
- Laws of large numbers and their applications
- Random processes
Additional information
Introduction to Probability - The Science of Uncertainty - Massachusetts Institute of Technology