Networks for learning: regression and classification
Master
In Maynard (USA)
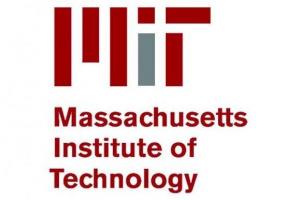
Description
-
Type
Master
-
Location
Maynard (USA)
-
Start date
Different dates available
During this course we will examine applications of several learning techniques in areas such as computer vision, computer graphics, database search and time-series analysis and prediction. Supervised learning with the use of regression and classification networks with sparse data sets will be explored. The extensive reading list grounds the future researcher in the field of learning networks. Lecture notes provide an overview of each topic covered in the class.
Facilities
Location
Start date
Start date
Reviews
Subjects
- Project
- Graphics
- Database training
- Database
- Networks
Course programme
Lectures: 2 sessions / week, 1.5 hours / session
The course focuses on the problem of supervised learning within the framework of Statistical Learning Theory. It starts with a review of classical statistical techniques, including Regularization Theory in RKHS for multivariate function approximation from sparse data. Next, VC theory is discussed in detail and used to justify classification and regression techniques such as Regularization Networks and Support Vector Machines. Selected topics such as boosting, feature selection and multiclass classification will complete the theory part of the course. During the course we will examine applications of several learning techniques in areas such as computer vision, computer graphics, database search and time-series analysis and prediction. We will briefly discuss implications of learning theories for how the brain may learn from experience, focusing on the neurobiology of object recognition. We plan to emphasize hands-on applications and exercises, paralleling the rapidly increasing practical uses of the techniques described in the subject.
18.02 (calculus) or permission of instructor.
Grades will be based on a nonlinear function:
f = f(Problem set 1, Problem set 2, Problem set 3, Final Project, attendance, interest, effort).
We may post data-based regression of the function after the fact, that is after grades are given. As a guideline, the final grade may be approximated by:
f ~ 0.3 Problem set 1 + 0.3 Problem set 2 + max [(Final Project), (Final Project + Problem set 3)]. But in this advanced graduate class, effort, interest, and attendance will also be taken into account!
Don't show me this again
This is one of over 2,200 courses on OCW. Find materials for this course in the pages linked along the left.
MIT OpenCourseWare is a free & open publication of material from thousands of MIT courses, covering the entire MIT curriculum.
No enrollment or registration. Freely browse and use OCW materials at your own pace. There's no signup, and no start or end dates.
Knowledge is your reward. Use OCW to guide your own life-long learning, or to teach others. We don't offer credit or certification for using OCW.
Made for sharing. Download files for later. Send to friends and colleagues. Modify, remix, and reuse (just remember to cite OCW as the source.)
Learn more at Get Started with MIT OpenCourseWare
Networks for learning: regression and classification