Quantitative Methods: Social Sciences
Course
In New York City (USA)
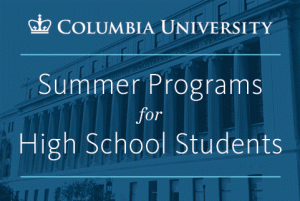
Description
-
Type
Course
-
Location
New york city (USA)
In this course you wil learn about: Data Analysis with Python, machine learning for the social sciences and modern data structures.
Facilities
Location
Start date
Start date
Reviews
This centre's achievements
All courses are up to date
The average rating is higher than 3.7
More than 50 reviews in the last 12 months
This centre has featured on Emagister for 5 years
Subjects
- Networks
- Data analysis
- Statistics
- Pythone
- Software
- Mathematics
- Machine Learning
- Algebra
- Calibration
- Matrix factorization
Course programme
- DATA ANALYSIS WITH PYTHON
This course is meant to provide an introduction to regression and applied statistics for the social sciences, with a strong emphasis on utilizing the Python software language to perform the key tasks in the data analysis workflow. Topics to be covered include various data structures, basic descriptive statistics, regression models, multiple regression analysis, interactions, polynomials, Gauss-Markov assumptions and asymptotics, heteroskedasticity and diagnostics, data visualization, models for binary outcomes, models for ordered data, first difference analysis, factor analysis, and cluster analysis. Through a variety of lab assignments, students will be able to generate and interpret quantitative data in helpful and provocative ways. Only relatively basic mathematics skills are assumed, but some more advanced math will be introduced as needed. A previous introductory statistics course that includes linear regression is helpful, but not required.
- MACHINE LEARNING FOR THE SOCIAL SCIENCES
Prerequisites: basic probability and statistics, basic linear algebra, and calculus This course will provide a comprehensive overview of machine learning as it is applied in a number of domains. Comparisons and contrasts will be drawn between this machine learning approach and more traditional regression-based approaches used in the social sciences. Emphasis will also be placed on opportunities to synthesize these two approaches. The course will start with an introduction to Python, the scikit-learn package and GitHub. After that, there will be some discussion of data exploration, visualization in matplotlib, preprocessing, feature engineering, variable imputation, and feature selection. Supervised learning methods will be considered, including OLS models, linear models for classification, support vector machines, decision trees and random forests, and gradient boosting. Calibration, model evaluation and strategies for dealing with imbalanced datasets, n on-negative matrix factorization, and outlier detection will be considered next. This will be followed by unsupervised techniques: PCA, discriminant analysis, manifold learning, clustering, mixture models, cluster evaluation. Lastly, we will consider neural networks, convolutional neural networks for image classification and recurrent neural networks. This course will primarily us Python. Previous programming experience will be helpful but not requisite. Prerequisites: basic probability and statistics, basic linear algebra, and calculus.
- MODERN DATA STRUCTURES
This course is intended to provide a detailed tour on how to access, clean, “munge” and organize data, both big and small. (It should also give students a flavor of what would be expected of them in a typical data science interview.) Each week will have simple, moderate and complex examples in class, with code to follow. Students will then practice additional exercises at home. The end point of each project would be to get the data organized and cleaned enough so that it is in a data-frame, ready for subsequent analysis and graphing. Therefore, no analysis or visualization (beyond just basic tables and plots to make sure everything was correctly organized) will be taught; and this will free up substantial time for the “nitty-gritty” of all of this data wrangling. The course will run for the 6-week duration of the Columbia Summer Session D, from May 28th through July 5th, 2019.
Quantitative Methods: Social Sciences